Alessandro Farbo
- 505306
- Phd: 38th cycle
- Department of Agricultural, Forest and Food Sciences
- Matriculation number: 804265
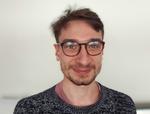
Phd thesis
Precision farming has emerged as a promising approach to optimize agricultural practices by tailoring
them to specific field conditions. This Ph.D. project focuses on leveraging the combined power of remote
sensing and artificial neural networks (ANNs) to enhance precision farming techniques, thereby enabling
more efficient resource management and improved crop yield. Remote sensing technologies, such as
satellite imagery and unmanned aerial vehicles (UAVs), offer valuable data on various crop-related
parameters, including vegetation indices, soil moisture content, and crop health indicators. By integrating
these remotely sensed data with ANNs, an intelligent system can be developed to analyze and interpret
the information in a comprehensive manner. ANNs are computational models inspired by the human
brain, capable of learning patterns and making predictions based on input data. The project aims to
develop an advanced framework that integrates remote sensing data and ANNs to provide real-time
monitoring and decision support for precision farming applications. The first step involves the collection
and preprocessing of remote sensing data from different platforms, ensuring the data's accuracy and
compatibility. Next, various ANN architectures, such as convolutional neural networks (CNNs) and
recurrent neural networks (RNNs), will be explored and optimized for specific farming tasks, such as crop
classification, disease detection, and yield prediction. Once the ANNs are trained and validated, they will
be deployed in a precision farming system, where they will continuously analyze the incoming remote
sensing data to provide actionable insights. The system will leverage the power of cloud computing to
process large volumes of data efficiently and generate near-real-time predictions. The proposed
framework has the potential to revolutionize precision farming practices by enabling farmers to make
data-driven decisions and optimize resource allocation. By reducing manual labor and optimizing
resource usage, precision farming can contribute to sustainable agriculture and mitigate environmental
impacts.
Research activities
Farbo, A., Sarvia, F., De Petris, S., Basile, V., Borgogno-Mondino, E., 2024. Forecasting corn NDVI through AI-based approaches using sentinel 2 image time series. ISPRS J. Photogramm. Remote Sens. 211, 244–261. https://doi.org/10.1016/j.isprsjprs.2024.04.011
Farbo, Alessandro, Meloni, R., Blandino, M., Sarvia, F., Reyneri, A., Borgogno-Mondino, E., 2022. Spectral Measures from Sentinel-2 Imagery vs Ground-Based Data from Rapidscan© Sensor: Performances on Winter Wheat, in: Borgogno-Mondino, E., Zamperlin, P. (Eds.), Geomatics for Green and Digital Transition. Springer International Publishing, Cham, pp. 211–221. https://doi.org/10.1007/978-3-031-17439-1_15
Farbo, A., Sarvia, F., De Petris, S., Borgogno-Mondino, E., 2022. PRELIMINARY CONCERNS ABOUT AGRONOMIC INTERPRETATION OF NDVI TIME SERIES FROM SENTINEL-2 DATA: PHENOLOGY AND THERMAL EFFICIENCY OF WINTER WHEAT IN PIEMONTE (NW ITALY). Int. Arch. Photogramm. Remote Sens. Spat. Inf. Sci. XLIII-B3-2022, 863–870. https://doi.org/10.5194/isprs-archives-XLIII-B3-2022-863-2022
Farbo, Alessandro, Trombetta, N.G., de Palma, L., Borgogno-Mondino, E., 2024. Estimation of Intercepted Solar Radiation and Stem Water Potential in a Table Grape Vineyard Covered by Plastic Film Using Sentinel-2 Data: A Comparison of OLS-, MLR-, and ML-Based Methods. Plants 13, 1203.
Borgogno-Mondino, E., Farbo, A., Novello, V., Palma, L. de, 2022. A Fast Regression-Based Approach to Map Water Status of Pomegranate Orchards with Sentinel 2 Data. Horticulturae 8, 759. https://doi.org/10.3390/horticulturae8090759
De Petris, S., Sarvia, F., Parizia, F., Ghilardi, F., Farbo, A., Borgogno-Mondino, E., 2024. Assessing mixed-pixels effects in vineyard mapping from Satellite: A proposal for an operational solution. Comput. Electron. Agric. 222, 109092. https://doi.org/10.1016/j.compag.2024.109092
Sarvia, F., De Petris, S., Farbo, A., Borgogno-Mondino, E., 2024. Geometric vs spectral content of Remotely Piloted Aircraft Systems images in the Precision agriculture context. Egypt. J. Remote Sens. Space Sci. 27, 524–531. https://doi.org/10.1016/j.ejrs.2024.06.003
Ghilardi, F., Petris, S.D., Farbo, A., Sarvia, F., Borgogno-Mondino, E., 2022. Exploring Stability of Crops in Agricultural Landscape Through GIS Tools and Open Data, in: Gervasi, O., Murgante, B., Misra, S., Rocha, A.M.A.C., Garau, C. (Eds.), Computational Science and Its Applications – ICCSA 2022 Workshops. Springer International Publishing, Cham, pp. 327–339. https://doi.org/10.1007/978-3-031-10545-6_23